Chhaya Kulkarni PhD Dissertation Defense
Thursdasy, April 24, 2025 at 10a [virtual]
We are excited to share that Chhaya Kulkarni (UMBC) will be defending her PhD Dissertation on Thursday, April 24, 2025 at 10a (est)
Date: Thursday, April 24, 2025
Time: 10a (est)
Webex Virtual Link: https://umbc.webex.com/meet/ckulkar1&sa=D&source=calendar&ust=1744638730592909&usg=AOvVaw0sVCREU9XCLE2gMztA2ojD
Title Multi-Contextual Learning in Spatiotemporal Neighborhoods
Committee
Dr. Vandana Janeja, Chair/Advisor
Dr. Jianwu Wang
Dr. Karuna Joshi
Dr. Bayu Adhi Tama
Dr. Nicole Schlegel
Abstract
This dissertation presents a multi-contextual learning framework within spatiotemporal neighborhoods to tackle analytical challenges in Earth observation data. The rapid rise in the amount of environmental data —as demonstrated by the European Space Agency's Copernicus program expanding from 2 to 20 petabytes per year and NASA's Earth Observing System producing 16 terabytes per day—poses difficulties to conventional analytical methods for managing multi-source data fusion, cross-scale pattern recognition, and spatial autocorrelation.
The work develops a context-aware spatiotemporal data analysis approach with a neighborhood-based spatiotemporal framework at its foundation. The framework employs Voronoi tessellation for micro-neighborhood generation and attribute-based grouping for macro-neighborhood generation. By incorporating contextual information from spatial proximity and attribute similarity, the approach captures nuanced patterns that traditional methods tend to ignore.
This multi-contextual learning framework is validated through two complementary application domains that serve as case studies. The Greenland Ice Sheet case demonstrates how the application of neighborhood analysis successfully encapsulates intricate melt behavior, accounting for local variability and interactions between temperature, albedo, and other variables. Digital twin simulations form a second test case by demonstrating that the same neighborhood-based approach is capable of delineating areas with analogous variance structures within high-resolution atmospheric data.
The methodological contributions of the dissertation are: (1) multi-contextual learning for spatiotemporal neighborhood formation; (2) Graph Deviation Networks for multivariate anomaly detection in such neighborhoods; (3) a comparative framework for the validity verification of data sources; and (4) spatial clustering for variance analysis. Each component tackles intrinsic challenges in spatiotemporal data analysis while offering practical solutions for environmental monitoring application scenarios. Results indicate that multi-contextual learning in spatiotemporal neighborhoods substantially enhances detection and interpretation capability for complex Earth observation data, with immediate implications for environmental monitoring, modeling, and satellite-based observational systems.
This dissertation presents a multi-contextual learning framework within spatiotemporal neighborhoods to tackle analytical challenges in Earth observation data. The rapid rise in the amount of environmental data —as demonstrated by the European Space Agency's Copernicus program expanding from 2 to 20 petabytes per year and NASA's Earth Observing System producing 16 terabytes per day—poses difficulties to conventional analytical methods for managing multi-source data fusion, cross-scale pattern recognition, and spatial autocorrelation.
The work develops a context-aware spatiotemporal data analysis approach with a neighborhood-based spatiotemporal framework at its foundation. The framework employs Voronoi tessellation for micro-neighborhood generation and attribute-based grouping for macro-neighborhood generation. By incorporating contextual information from spatial proximity and attribute similarity, the approach captures nuanced patterns that traditional methods tend to ignore.
This multi-contextual learning framework is validated through two complementary application domains that serve as case studies. The Greenland Ice Sheet case demonstrates how the application of neighborhood analysis successfully encapsulates intricate melt behavior, accounting for local variability and interactions between temperature, albedo, and other variables. Digital twin simulations form a second test case by demonstrating that the same neighborhood-based approach is capable of delineating areas with analogous variance structures within high-resolution atmospheric data.
The methodological contributions of the dissertation are: (1) multi-contextual learning for spatiotemporal neighborhood formation; (2) Graph Deviation Networks for multivariate anomaly detection in such neighborhoods; (3) a comparative framework for the validity verification of data sources; and (4) spatial clustering for variance analysis. Each component tackles intrinsic challenges in spatiotemporal data analysis while offering practical solutions for environmental monitoring application scenarios. Results indicate that multi-contextual learning in spatiotemporal neighborhoods substantially enhances detection and interpretation capability for complex Earth observation data, with immediate implications for environmental monitoring, modeling, and satellite-based observational systems.
Tags:
Posted: April 17, 2025, 3:30 PM
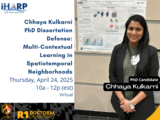