Francis Nji successfully defends his PhD Proposal
Congratulatoins Francis!
Francis Nji, iHARP Research Assistant successfully defended his PhD Proposal on Monday, January 27, 2025. Join iHARP in congratulating Francis on his successful PhD Proposal defense!
Title
Accurate Clustering of Multi-dimensional Multivariate Spatiotemporal data
Committee
- Dr Jianwu Wang - Advisor and Committee Chair (UMBC/ iHARP)
- Dr Vandana Janeja - Co-advisor and Committee Member (UMBC/ iHARP)
- Dr Aneesh Subramanian - Committee Member (UC-Boulder / iHARP)
- Dr James Foulds - Committee Member (UMBC)
- Dr Yiqun Xie - Committee Member (UMD)
Abstract
The growing availability of multivariate spatiotemporal data, which
includes datasets containing both spatial and temporal dimensions across
multiple variables, presents significant opportunities for extracting
insights into complex environmental systems, societal trends, and
dynamic processes in fields such as environmental monitoring, urban
planning, traffic management, transportation, social media analysis,
epidemiology, climatology, crime analysis and disaster management where
understanding the interactions between spatial locations and their
evolution over time is crucial for decision-making. Proper analysis of
these datasets enable researchers to understand interactions and
patterns that evolve over time and space, facilitating advancements in
predictive modeling, causal analysis, and decision-making for addressing
global challenges like climate change, resource management, and public
health crises. One of such analytical approaches to extract meaningful
insights from this data is clustering. Clustering is the process of
grouping data with similar spatial attributes, temporal attributes, or
both, from which many significant events and regular phenomena can be
discovered. However, clustering this data is highly challenging due to
the complexities involved in accounting for both spatial autocorrelation
and temporal dependencies, as well as the high dimensionality of
multivariate data. To tackle these challenges, this dissertation
presents three innovative approaches aimed at accurately partitioning
complex multivariate spatiotemporal data such that similar points are
grouped together and dissimilar points are segregated. Each proposed
model is designed to capture the nuanced spatial and temporal
relationships inherent in the data, while enhancing clustering
performance and stability. By leveraging advanced traditional and deep
learning techniques, the proposed models provide robust solutions for
managing the complexities of spatiotemporal datasets, resulting in more
accurate, stable and interpretable clustering outcomes.The first proposed model, Hybrid Ensemble Deep Graph Temporal Clustering (HEDGTC), integrates homogeneous and heterogeneous ensemble clustering techniques in an attempt to harness their individual strengths while mitigating their weaknesses. HEDGTC further employs a dual-consensus approach to address noise and misclassification that might result from base clusters. To obtain the desired clusters, HEDGTC employs a deep graph attention autoencoder network which simultaneously updates the clustering loss and reconstruction loss to improve the clustering results in terms of performance and stability. When compared with existing state-of-the-art ensemble models, HEDGTC outperforms with significant margins proving capable to capture implicit temporal patterns and provides consistent results when tested on real-world multivariate spatiotemporal datasets. Although HEDGTC outperforms existing ensemble algorithms, it has its limitations. Real-world multivariate spatiotemporal data is truly complex and can be characterized by non-linearity: variables may exhibit nonlinear interdependencies, localized patterns: clusters may form in specific regions of space, time, or feature combinations, irrelevant dimensions: datasets often contain redundant information, or irrelevant variables and overlapping clusters: a single data point can belong to different clusters. In such cases, HEDGTC might have a hard time to deal with this dependencies therefore the need to develop advanced algorithms that, unlike HEDGTC which rely on global features to perform clustering, will include local feature subspaces and the capability to capture underlying structures in data with both spatial and temporal dimensions.
To address the limitations of HEDGTC, we propose a novel Attention-Guided Deep Temporal Subspace Clustering (A-DATSC) for multivariate spatiotemporal data. A-DATSC incorporates a deep subspace clustering generator and a quality-verifying discriminator that work in tandem. Inspired by the recent success of the U-Net architecture, the generator combines CNN-RNN-attention mechanisms in an autoencoder to capture spatial, temporal and salient representations respectively present in multivariate spatiotemporal data. The autoencoder is equipped with a fully connected GNN-based self-expressive network that extracts the weights of the latent features into a coefficient matrix and a clustering layer that performs clustering through the optimization of the reconstruction loss, self-expressive loss and clustering loss in a iterative manner. The discriminator evaluates current clustering performance by inspecting whether the re-sampled data from estimated subspaces have consistent subspace properties, and supervises the generator to progressively improve subspace clustering. Experimental results on three real-world multivariate spatiotemporal data demonstrate the advantages of A-DATSC over shallow and few deep subspace clustering models.
In recent years, research on clustering analysis has largely focused on improving accuracy and efficiency, often at the cost of interpretability. Geospatial clustering of multivariate spatiotemporal data plays a critical role in analyzing complex spatial patterns for applications such as urban planning, mobility analysis, and climate monitoring. However, the interpretability of clustering results remains a significant challenge due to the "black-box" nature of clustering algorithms and the inherent complexity of multivariate spatiotemporal data. Ensuring interpretability is essential for fostering trust, meeting ethical standards, and complying with regulatory requirements, as clustering-derived decisions must be transparent and justifiable. To address these challenges, we propose a novel end-to-end Interpretable Causal Clustering (ICC) model for high dimensional multivariate spatiotemporal data. ICC employs a causal-discovery feature engineering pre-clustering and a causal inference in-clustering phase. Pre-clustering is achieved through an ensemble of causal discovery methods to prioritize causally significant features, enhanced by spatial modeling and sparsity regularization to focus on relevant features. In-clustering is achieved through a U-Net Autoencoder architecture with stacked GATv2 layers for capturing spatial dependencies and ConvLSTM for temporal modeling. ICC integrates a Probabilistic Discriminative Model (PDM) at the latent encoding layer to further enhance the encoding of causally significant features, ensuring that the latent representations respect causal constraints. ICC incorporates Dynamic Bayesian Networks as a causal inference technique to ensure that the clustering process respects causal dependencies. To improve clustering results, ICC introduces a causal regularization loss term that penalizes clusters that violate causal constraints. To further enhance interpretability, ICC introduces Counterfactual reasoning that seeks to validate clusters for causal consistency and maps them onto geospatial and temporal causal graphs. This further tests the validity of the clusters if they reflect true causal relationships. ICC mitigates confounding effects by explicitly modeling confounders which reduces noise and spurious correlations. Experimental results demonstrate that ICC significantly enhances interpretability and accuracy in geospatial clustering, offering actionable insights into the dynamics of multivariate spatiotemporal climate data. We plan to evaluate our approach on a suite of synthetic and real world clustering problems, and compare across state of the art interpretable and non-interpretable clustering algorithms.
Tags:
Posted: January 28, 2025, 2:01 PM
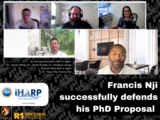