Akila Sampath successfully defends her PhD Proposal
Congratulatoins Akila!
Akila Sampath, iHARP Research Assistant, successfully defended her PhD
Proposal on Wednesday, December 4, 2024. Join iHARP in congratulating Akila on her successful PhD proposal defense.
Title
Leveraging Physical Principles in Deep Learning to Study Arctic Sea Ice
Committee
- Dr. Jianwu Wang, Chair/Advisor (UMBC)
- Dr. Vandana Janeja, Co-Chair (UMBC)
- Dr. Houbing Song (UMBC)
- Dr. James Foulds (UMBC)
- Dr. Donald.k.Perovich (Dartmouth College)
- Dr. Nicole Schlegel (NOAA)
Abstract
The Arctic region is undergoing rapid environmental changes, with sea
ice loss being a prominent indicator. Accurate prediction of sea ice
thickness and extent is crucial for understanding climate change impacts
and informing policy decisions. Traditional climate models often
struggle to capture the complex interactions between atmospheric,
oceanic, and sea ice processes. In recent years, machine learning has
emerged as a powerful tool for analyzing large datasets and making
accurate predictions. However, machine learning models, while capable of
capturing complex patterns, can sometimes produce unrealistic or
physically implausible results. To address this limitation, we propose a
novel approach that combines the power of machine learning with the
rigor of physical principles. By integrating physics-informed techniques
into machine learning models, we aim to develop more accurate and
reliable predictions of sea ice conditions.
Physics-Informed Machine
Learning (PIML) is a novel approach that blends traditional scientific
models with the pattern-recognition capabilities of machine learning to
improve predictions of sea ice thickness. By leveraging both data-driven
and physics-based knowledge, PIML offers more accurate and reliable
predictions, even in data-scarce environments. This approach is
particularly well-suited for studying complex systems like the Arctic
sea ice. Our research introduces Physics-Encoded Neural Networks (PeNNs)
to predict snow density. PeNNs embed physical laws directly into the
neural network architecture, enabling the estimation of hidden physical
properties from observable data. This makes PeNNs highly effective for
tracking snow and ice conditions with precision. Additionally, we
developed a physics-guided model (PGM) to investigate the causal link
between sea ice loss and increased turbulence in the Beaufort Gyre
through wind current. By incorporating physical constraints, the PGM
ensures that predictions align with known scientific principles, aiding
in the understanding of these causal relationships. Our proposed models
offer a more comprehensive approach to studying Arctic sea ice. By
integrating physics into machine learning, we aim to create tools that
are both accurate and interpretable. This research provides valuable
insights into Arctic climate change and demonstrates how physics can
enhance the capabilities of machine learning models, leading to a deeper
understanding of the impacts of sea ice loss.
At the top pictured is Akila Sampath,
Middle Row, left to right: Dr. Jianwu Wang, Dr. Vandana Janeja, Dr.Nicole Schlegel. Bottom Row, left to right: Dr. Donald.k.Perovich, Dr. James Foulds, Dr. Dr. Houbing Song
Tags:
Posted: December 10, 2024, 4:09 PM
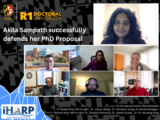