Sahara Ali PhD Dissertation Defense
Thursdasy, June 6, 2024 at 2p [virtual]
We are excited to share that Sahara Ali will be defending her PhD
Dissertation on Thursday, June 6 at 2p (est).
PhD Candidate: Sahara Ali
Dissertation Title: Spatiotemporal Forecasting and Causality Methods for the Arctic Amplification
Committee:
Dissertation Title: Spatiotemporal Forecasting and Causality Methods for the Arctic Amplification
Committee:
- Dr Jianwu Wang (Committee Chair, UMBC)
- Dr Vandana Janeja (UMBC)
- Dr Sanjay Purushotham (UMBC)
- Dr Md. Osman Gani (UMBC)
- Dr Aneesh Subramanian (UC Boulder)
- Dr Yiyi Huang (UMBC / Bloomberg)
Date and Time: June 6th, 2024, 2-4pm EST
Date and Time: June 6th, 2024, 2-4pm EST
Meeting Information:
Meeting link:
https://umbc.webex.com/umbc/j.php?MTID=me7c6a976cb64159dd2cf7944e72a250a
Meeting number:
2632 192 0478
Meeting password:
gxF8cQCRT77
Join by phone
+1-202-860-2110 United States Toll (Washington D.C.)
Access code: 26321920478
Global call-in numbers
https://umbc.webex.com/umbc/globalcallin.php?MTID=m922682770606a3cedacc1779cee0a25f
https://umbc.webex.com/umbc/j.php?MTID=me7c6a976cb64159dd2cf7944e72a250a
Meeting number:
2632 192 0478
Meeting password:
gxF8cQCRT77
Join by phone
+1-202-860-2110 United States Toll (Washington D.C.)
Access code: 26321920478
Global call-in numbers
https://umbc.webex.com/umbc/globalcallin.php?MTID=m922682770606a3cedacc1779cee0a25f
Abstract:
The
Arctic is a region with unique climate features where warming has been
almost twice as fast as the rest of the world. The warming of the
Arctic, also known as Arctic amplification, is led by several
atmospheric and oceanic drivers. The essential part of Arctic
amplification is the unprecedented sea ice loss as demonstrated by
satellite observations. However, understanding the causes of sea-ice
variations and its feedback on the atmospheric processes is a complex
task. Therefore, accurately forecasting Arctic sea ice from sub-seasonal
to seasonal scales has been a major scientific challenge and has gained
interest of Data Scientists to develop novel statistical and machine
learning approaches for this task. This brings us to three key research
areas studied in this dissertation: (i) accurate forecasting of climate
data in the Arctic on sub-seasonal to seasonal scales, (ii) estimating
the influence of atmospheric processes and their time-varying effects on
the sea-ice and ice-sheet variations. (iii) estimating the spatial
interference of atmospheric processes and their time-varying effects on
the sub-regional sea-ice variations.
For the first topic, this
research explores the potential of data-driven approaches to study sea
ice variations by proposing custom deep learning modeling techniques to
learn spatiotemporal variations in the sea ice. By combining the spatial
skills of convolutional neural networks (CNNs), predictive power of
recurrent neural networks (RNNs) and other hybrid modeling techniques
(ConvLSTM), the proposed models reduce the prediction error by 60% as
compared to the state-of-the-art approaches. The work further
contributes to accurate long-term forecasting beyond the seasonal
barrier.
For the second topic, the research utilizes potential
outcome framework to infer causation in climate data on daily and
monthly temporal scales using custom deep learning based forecasting
models. In comparison with related work, the framework proposes a deep
learning based causal inference model to infer causation under
continuous treatment using recurrent neural networks, and a novel
probabilistic balancing technique to reduce the confounding bias.
For the third topic, this work formalizes the concept of spatial interference in case of time-varying treatment assignments by extending the potential outcome framework under the assumption of no unmeasured confounding. The extended framework utilizes latent factor modeling to reduce the bias due to time-varying confounding while leveraging the power of U-Net architecture to capture global and local spatial interference in data over time. The proposed causal estimators are an extension of average treatment effect (ATE) for estimating direct (DATE) and indirect effects (IATE) of spatial interference on treated and untreated data. Being the first of its kind deep learning based spatiotemporal causal inference technique, the proposed approach shows advantages over several baseline methods based on the experiment results on synthetic datasets, with and without spatial interference. The empirical results on the Arctic dataset not only align with domain knowledge, but also pave paths in quantifying the impact of causal drivers of climate change in the Arctic.
For the third topic, this work formalizes the concept of spatial interference in case of time-varying treatment assignments by extending the potential outcome framework under the assumption of no unmeasured confounding. The extended framework utilizes latent factor modeling to reduce the bias due to time-varying confounding while leveraging the power of U-Net architecture to capture global and local spatial interference in data over time. The proposed causal estimators are an extension of average treatment effect (ATE) for estimating direct (DATE) and indirect effects (IATE) of spatial interference on treated and untreated data. Being the first of its kind deep learning based spatiotemporal causal inference technique, the proposed approach shows advantages over several baseline methods based on the experiment results on synthetic datasets, with and without spatial interference. The empirical results on the Arctic dataset not only align with domain knowledge, but also pave paths in quantifying the impact of causal drivers of climate change in the Arctic.
Overall, these
predictive and inferential models have the potential to generalize for
multiple downstream tasks and can be extended to other domains beyond
Earth Science.
Tags:
Posted: May 28, 2024, 9:56 AM
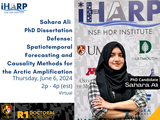