Tolulope Ale successfully defends his PhD Proposal
Congratulations Tolulope!
Tolulope Ale, iHARP Research Assistant, successfully defended his PhD Proposal on Thursday, May 23, 2024. Join iHARP in congratulating Tolulope on his
successful PhD proposal defense.
In response, we propose a novel framework incorporated with feature attribution for detecting anomalies within multivariate time series data and then analyzing climate change trends in polar regions. Our methodology employs a Variational Autoencoder (VAE) framework, chosen for its stochastic nature, which we enhanced by incorporating correlation-based feature clustering and dynamic thresholding. These enhancements allow the VAE to focus on localized representations, thereby enriching the latent representation quality and the accuracy of detected anomalies. Given the complex interdependencies among variables and over time within multivariate climate data, we introduce the concepts of temporal overlap and proximity. These concepts allow us to identify how an anomaly in a variable relates to an anomaly in other variables. Through extensive experimentation on three distinct datasets, our research substantiates the efficacy of the proposed framework, marking a significant advancement in anomaly detection within climate data analysis
Title: A Framework for Multivariate Explainable Anomaly Detection with Uncertainty Estimation for Time Series Data.
Committee:
Dr. Vandana Janaja - Advisor and Committee Chair (UMBC)
Dr. Nicole-Jeanne Schlegel - Committee Member (NOAA)
Dr. Jianwu Wang - Committee Member (UMBC)
Dr. Patti Ordóñez - Committee Member (UMBC)
Dr. Sudip Chakraborty - Committee Member (UMBC)
Abstract:
The
multivariate time series analysis within climate data represents a
crucial yet less explored field. This is particularly relevant when
examining extreme climate events, such as snow melting in polar regions,
which necessitate the consideration of multiple variables to capture
fluctuations accurately. Anomalies in climate data often result from the
interplay of several variables, meaning that what appears anomalous
under univariate analysis may, in fact, align with expected patterns
once contextualized within a multivariate framework. This approach more
accurately reflects the interconnected nature of real-world phenomena,
where events seldom occur in isolation. The primary challenge in anomaly
detection algorithms for multivariate climate time series lies in
effectively harnessing the intricate relationships among the
multivariate data. Despite the advancements in deep learning for anomaly
detection, very few efforts have been directed toward climate data;
this could be due to the lack of labeled data and the complexity of
climate variables. Additionally, a significant limitation of existing
anomaly detection algorithms is their lack of explainability, especially
in climate data, where it is crucial to pinpoint which variable most
significantly influences an anomaly score. Beyond merely identifying
anomalies, it is vital to ascertain the primary variable contributing to
the anomaly, facilitating targeted strategies for mitigating such
occurrences in climate datasets.
In response, we propose a novel framework incorporated with feature attribution for detecting anomalies within multivariate time series data and then analyzing climate change trends in polar regions. Our methodology employs a Variational Autoencoder (VAE) framework, chosen for its stochastic nature, which we enhanced by incorporating correlation-based feature clustering and dynamic thresholding. These enhancements allow the VAE to focus on localized representations, thereby enriching the latent representation quality and the accuracy of detected anomalies. Given the complex interdependencies among variables and over time within multivariate climate data, we introduce the concepts of temporal overlap and proximity. These concepts allow us to identify how an anomaly in a variable relates to an anomaly in other variables. Through extensive experimentation on three distinct datasets, our research substantiates the efficacy of the proposed framework, marking a significant advancement in anomaly detection within climate data analysis
Image Note: In the left panel, Naomi Tack is defending her dissertation. In the right panel, Naomi stands with her iHARP mentors, (left to right: Dr. Becca Williams, Naomi Tack, and Dr. Don Engel).
Photo to the left: Pictured is Tolulope Ale defending his proposal.
Photo to the right: Pictured is Tolulope Ale standing with his committee. In the photo: Left of screen Left to Right: Dr. Vandana Janeja, Tolulope Ale
On Screen Top Right: Dr. Jianwu Wang, Bottom Left: Dr. Sudip Chakraborty, Bottom Right: Dr. Nicole-Jeanne Schegel
Right of Screen: Dr. Patti Ordóñez
Tags:
Posted: May 24, 2024, 3:15 PM
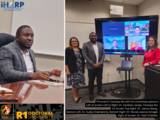