Sahara Ali Successfully Defends Thesis Proposal
Sahara Ali successfully defended her thesis proposal on Thursday, December 15! Help us celebrate her success and successful defense.
Congratulations Sahara!!!
Research Title
Spatiotemporal Forecasting And Causality Methods For Arctic Amplification
Abstract
Over the last three decades, the warming of the Arctic sea ice has been almost twice as fast as the rest of the world. This phenomenon, known as Arctic amplification, is caused by several atmospheric and thermodynamic processes. If the amplification continues at the same rate, it is feared that this amplification will further alter the climate patterns across the globe and lead to extreme weather events. Understanding the causes of sea-ice variations and its feedback on the atmospheric processes is a complex task. The first step in studying such a problem is the development of holistic causal graphs that can represent the underlying causal mechanisms of Arctic’s thermodynamic system. Once this graph is discovered, the next step is to estimate the influence of causal effect on a spatiotemporal scale; however, this cannot be accomplished in the presence of confounders varying both, spatially and temporally. This brings us to three key research areas: (i) accurate forecasting of sea ice on sub-seasonal to seasonal scales, (ii) combining results from multiple causal discovery methods to generate holistic aggregated causal graphs (iii) estimating the influence of atmospheric processes and their space-time-varying effects on Arctic sea-ice variations.
To cater to these research directions, this work proposes techniques for developing custom deep learning models to learn spatiotemporal variations in sea ice, followed by the development of a potential outcome framework to infer causation in climate data on both spatial and temporal scales using our forecasting models. Since the ground truth information is not available, the study further proposes a method to perform unsupervised ensembling to combine causal graphs discovered from existing methods. We believe our research will not only contribute to data-driven study of the Arctic but will also promote local communities and the broader international society to respond and adapt to a changing Arctic, thus having far-reaching impacts on forecasting global food and water demands, and economic security across the globe.
To cater to these research directions, this work proposes techniques for developing custom deep learning models to learn spatiotemporal variations in sea ice, followed by the development of a potential outcome framework to infer causation in climate data on both spatial and temporal scales using our forecasting models. Since the ground truth information is not available, the study further proposes a method to perform unsupervised ensembling to combine causal graphs discovered from existing methods. We believe our research will not only contribute to data-driven study of the Arctic but will also promote local communities and the broader international society to respond and adapt to a changing Arctic, thus having far-reaching impacts on forecasting global food and water demands, and economic security across the globe.
Committee Members
Dr. Jianwu Wang (Chair/Advisor), Dr. Vandana Janeja, Dr. Sanjay Purushotham, Dr. Md Osman Gani, Dr. Aneesh Subramanian, Dr. Yiyi Huang
Tags:
Posted: December 16, 2022, 11:31 AM
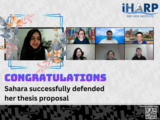